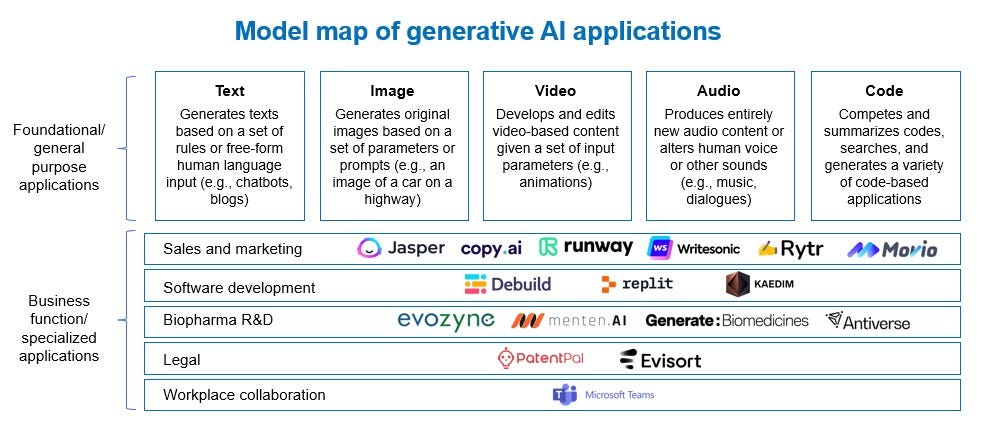
Peering into the Future: A Glimpse at Generative AI’s Role in Healthcare
Peering into the Future: A Glimpse of Generative AI’s Role in Healthcare
Artificial intelligence (AI) has always promised to bring about revolutionary changes in the future. The advancements in generative AI are now turning that potential into reality, garnering widespread interest and attention worldwide. Within two months of its launch, ChatGPT had already registered 100 million users, setting a record for the fastest-growing base of users.
While traditional AI reads through historical data to identify patterns and insights, generative AI goes beyond that. It still entails models built on vast quantities of data, but it uses that information to generate new content such as text, media (video, images, and speech), computer-aided design, and code.
Source: L.E.K.
In recent times, with an increasing incidence of pandemics, a surge in chronic diseases, mental health concerns, and a rapidly aging population, the healthcare industry has been struggling to keep up with the rising demand for high-quality care services. Studies have revealed that administrative factors account for as much as 50% of medical errors in primary care and the existing shortage of healthcare professionals worldwide exacerbates these issues.
Generative AI in Healthcare is a Beacon of Hope
Generative AI has immense potential to revolutionize various sub-segments in healthcare.
Generative AI is expected to have a total accessible market of US$121 billion by 2027 and is expected to grow at the fastest pace in healthcare compared to any other industry, with a compound annual growth rate of 85% through 2027.
Source: BCG
Presently, Generative AI is finding significant applications in two sub-segments within healthcare.
The first area is admin tasks within hospitals and clinics,
AI is being employed to automate various admin tasks, such as scheduling appointments, managing EHRs, note-taking, and streamlining billing. For e.g., generative AI copilots (co-doctors) that help doctors zoom through tasks like note-taking are proliferatingThese copilots assist doctors by automating tedious tasks like reading patient information and generating medical reports
The second area is in the early stages of healthcare delivery,
AI is being utilized for tasks like initial triage, preliminary diagnosis, and risk assessment. AI models can analyze patient data, symptoms, and medical history to provide suggestions or insights to healthcare professionals, aiding in decision-making during the early stages of patient interactionFor e.g., Ezra, a New York-based startup aims to help doctors detect cancer earlier. Its AI tool makes MRIs faster and cheaper and screens for cancer in organs that radiologists cannot currently screen forSource: About Digital Health
However, as the complexity of treatment increases, the involvement of AI tends to decrease. While AI can assist in diagnosing common or straightforward conditions, specialized medical cases often require the experience, skill, and judgment of highly trained surgeons. AI can be valuable in providing decision support and augmenting surgical procedures, but human expertise remains crucial in making critical decisions during complex surgical interventions.
Note: This is not an exhaustive list; Source: DC News Now, Healthark Insights
Evolving Role of AI in Critical Stages of Treatment
Source: Internal Analysis
Over the years, humans have accepted technologies they trust. For any technology, there has to be a recognition and acceptance of its value. It is imperative to develop and demonstrate the technical capabilities of generative AI in healthcare and effectively communicate its value.
Medicine has been a trust-based practice and generative AI for its widespread adoption has to gain the common mass’s trust. And more the criticality, more is the trust required. The current uses of generative AI in streamlining administrative tasks and in aiding in the early-stage prevention of illnesses are the low-hanging fruits of entering the healthcare value chain. But gaining trust in the critical stages will depend on 3 key factors:
Empirical backing — Should have empirical evidence supporting its effectiveness and demonstrating its ability to provide meaningful solutions or insightsRegulations — Impact and consequences of generative AI on society are still unfolding and require examination, and hence, earning regulatory approvals would be the first step in gaining trustTargeted use cases — Needs to be able to develop targeted use cases that demonstrate resilience to different scenarios and have measures in place to prevent and recover from any failures that might occur
Applications Across the Healthcare Value Chain
40% of all working hours in healthcare could be supported or augmented by language-based artificial intelligence, according to some estimates.
Moreover, 98% of healthcare providers agree that advancements in generative AI are ushering in a new era of enterprise intelligence.
Source: TLGG Group
Let’s quickly delve into the applications stated in the timeline chart:
Administrative tasks: Will help healthcare providers streamline the patient services process by automating many of the manual and time-consuming tasksMedical image enhancement: Will help doctors detect diseases at an early stage and make better decisions by cleaning and analyzing medical images like X-rays and MRIsPersonalized treatment plans: Will analyze large sets of data, such as medical history and lifestyle choices, and make predictions about how an individual will respond to a treatmentVirtual nursing assistant: Will assist HCPs in delivering quality care remotely by enhancing patient monitoring, medication reminders, and personalized health educationDrug discovery and development: Will make the research process faster and cost-effective; researchers can generate and analyze huge sets of molecular data to find potential drug candidates which would help in identifying promising compounds more quicklyPrecision medicine: Will bring in personalized medicines by analyzing genetic profiles and patient-specific information to predict disease outcomesRobotic surgery assistance: Will provide real-time assistance and decision support to a surgeon by analyzing surgical data, imaging, and patient information to improve precisionProsthesis designing: Similar to drug designing, generative AI will be used to make prostheses that would better fit the patients since they would be customized for themDisease prevention: May lead to a point where it can analyze vast datasets to identify patterns and risk factors, hence will enable early disease prediction and prevention strategiesAI-based HCPs: May advance to a point where AI-based healthcare providers autonomously diagnose and treat patientsBrain-computer interfaces: May allow direct communication between the brain and external devices, aiding in the management of neurological conditions such as Parkinson’s diseaseOrgan regeneration: May develop personalized regenerative therapies by analyzing cellular data which have the potential to revolutionize treatments for organ failurePharmacogenomics: Will play a crucial role in this field which focuses on understanding how genetic variations impact an individual’s response to medications
Confronting the Challenges Involved
While generative AI holds promise in addressing these challenges, it is crucial to acknowledge that this technology is still developing and presents its own obstacles.
Interpretability: Content produced by generative AI models may lack clarity, making it difficult to understand how the algorithm arrived at a specific resultAvailability of large and diverse datasets: Models rely on extensive datasets for training, and obtaining such datasets in healthcare can be challenging. Limited access to comprehensive and representative data can hinder the model’s ability to generate meaningful outputsOver-reliance: There exists the risk that healthcare providers may become overly reliant on these algorithms and lose the ability to make independent judgmentsPrivacy and security: Patient privacy is strictly regulated. The use of generative AI in healthcare raises concerns about protecting patient privacy, sensitive medical data, and the potential for misuse or unauthorized access to healthcare dataEthical considerations: The use of generative AI in healthcare raises several ethical concerns, such as the potential impact on employment in the healthcare sector
While certain projections indicate that generative AI has the potential to boost global GDP by 7% over a decade, its impact extends far beyond mere economic benefits. The rise of generative AI truly presents a transformative opportunity.